Thought Leadership
Innovation doesn’t happen in a vacuum. The following reflects some of the most important insights that have driven our advancements.
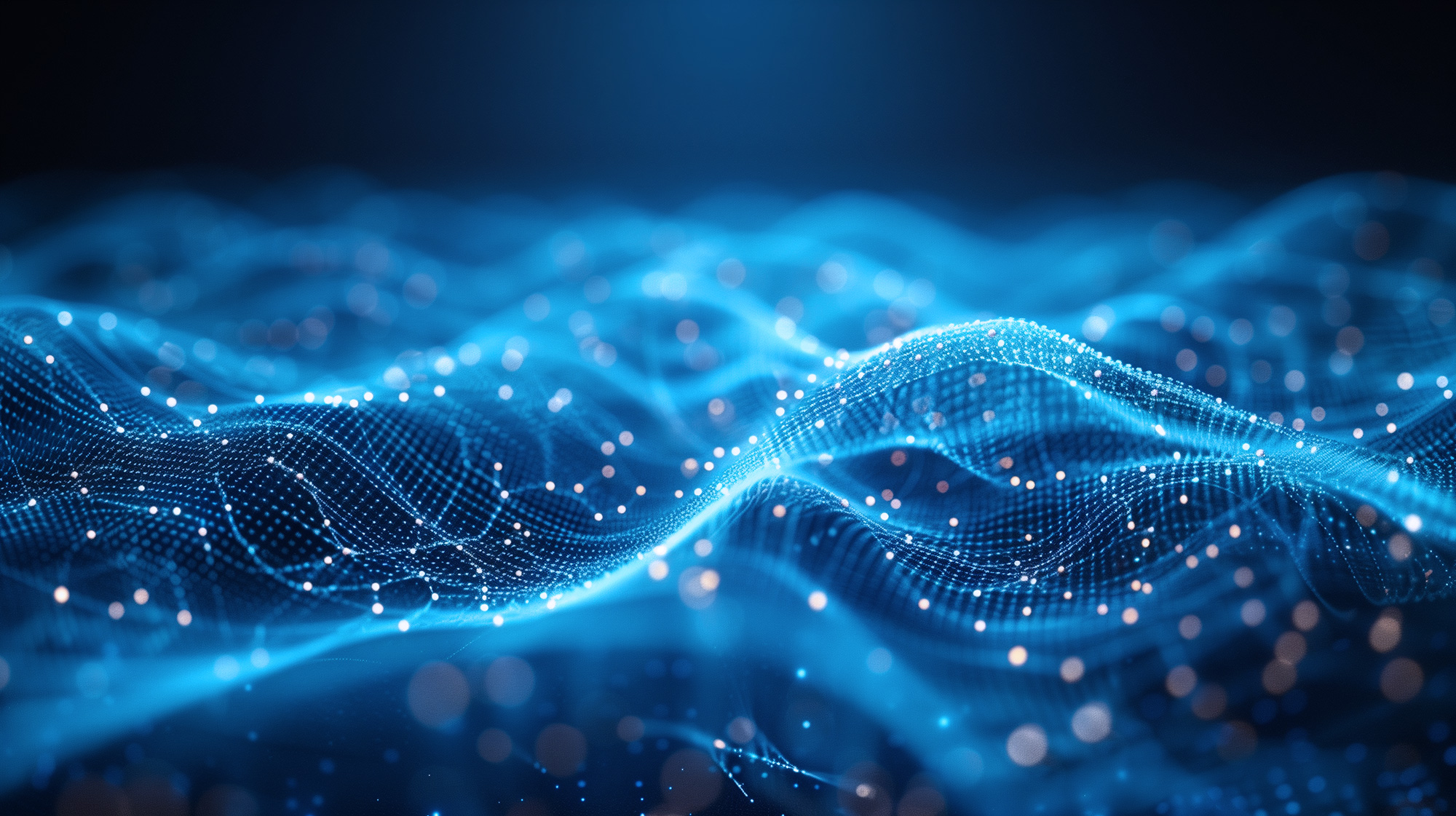
Foundational Research and Papers
EAM: How and Why AI-Powered Active Management Will Dominate Passive
This paper, edited and published by the CFA Institute, represents a comprehensive and thorough coverage of Ensemble Active Management (EAM) from design to performance validation. Key definitional sections include a description of EAM construction as well as the importance and impact of the Machine Learning concept known as Ensemble Methods. The paper also reviews summary relative performance data of EAM portfolios versus standard benchmarks as detailed in the largest academic study for EAM ever conducted (the 2024 EAM White Paper: Ensemble Active Management: AI's Transformation of Active Management), and finally presents performance data from live EAM portfolios that are active in the marketplace.
Ensemble Active Management: AI's Transformation of Active Management (2024 White Paper)
This seminal White Paper presents the results of the largest academic study of EAM ever conducted, representing multi-year performance of 60,000 EAM portfolios. The study was based on more than 330 mutual funds, from 140 fund firms, and representing $3 trillion in assets under management. Key results presented show how EAM portfolios structurally and persistently outperform both standard industry benchmarks and traditional actively managed mutual funds, while also reducing negative “tail risk” versus traditional mutual funds.
Capturing Ensemble Alpha
This paper, edited and published by the Investment & Wealth Institute, documents the development and validation of Ensemble Active Management (EAM). At its core, EAM represents the advancement of long practiced machine learning processes known as ensemble methods to address one of the most intractable challenge in investment management – reliably beating the relevant benchmark. This paper traces the origins of EAM’s development and the robust evidence of its efficacy, as well as its growing use.
Ensemble Active Management: The Blueprint for Rescuing Active Management
This white paper, edited and published by the CFA Institute, introduces Ensemble Active Management (EAM) as a transformative solution for the challenges of traditional active management. EAM integrates diverse active strategies through machine learning and advanced data analysis, aiming to enhance returns while minimizing risk. By systematically combining multiple approaches, EAM offers a more resilient framework for achieving alpha and managing portfolio volatility, addressing the shortcomings of traditional methods and paving the way for a more robust active management paradigm.
The Active Manager Paradox: High-Conviction Overweight Positions
This white paper, edited and published by the CFA Institute, provides groundbreaking insights into the performance of active managers. Through a comprehensive analysis of over 100 US equity funds, the research demonstrates that the only significant source of stock selection alpha comes from high conviction overweight positions. These positions, when isolated, outperformed their benchmarks in 83.7% of rolling one-year periods, even after accounting for fees. The study underscores the necessity for active managers to concentrate on their highest conviction stocks to achieve consistent outperformance, challenging the traditional portfolio design that dilutes potential returns.
Ensemble Active Management (EAM): Taming Toxic Tails
This paper, edited and published by the CFA Institute, details how EAM portfolios solve the “Active Manager’s Dilemma.” This dilemma, which structurally impairs most traditional, actively managed investment portfolios, reflects the conflict that exists when managers desire to build investment portfolios that are concentrated in their highest conviction stock selections (an approach proven to result in improved absolute performance) but are then subjected to increased risk of significant performance failures (or “Toxic Tails”).
Ensemble Active Management: The Next Evolution In Active Investment Management (2018 White Paper)
This White Paper explores Ensemble Active Management (EAM), a transformative approach that combines AI and traditional active management techniques. By leveraging multiple high conviction stock selections from different managers, EAM portfolios achieve superior performance and reduced risk. The study, based on 165 million data points, shows EAM portfolios outperforming the S&P 500 in 72% of rolling 1-year periods and 94% of rolling 3-year periods, delivering higher returns with greater reliability and risk-adjusted performance.
Related Academic Papers
Methodology, Design, and Data Integrity Validation Study of Turing Technology’s 2024 Ensemble Active Management White Paper
This is an independent report written after a months' long academic evaluation of the 2024 EAM White Paper "Ensemble Active Management: AI's Transformation of Active Management." Professor Goldsman and his team were granted complete transparency to the White Paper's methodology, stated biases, input and output data, and even code. They rebuilt the key results from the White Paper using alternative analytical and sampling techniques, validating the published results of the White Paper.
Mathematical Foundation for Ensemble Machine Learning and Ensemble Portfolio Analysis
This academic paper delves into the mathematical foundations of Ensemble Portfolio Analysis, demonstrating how combining high conviction picks from multiple managers enhances portfolio performance. By using ensemble voting and machine learning techniques, the approach significantly reduces error probability and boosts returns while managing risks. The paper illustrates that ensemble portfolios achieve higher Sharpe ratios compared to traditional methods, offering a robust framework for active management that consistently outperforms the market.